AI in water management: Balancing innovation and consumption | White & Case LLPCommunities across the globe are facing water scarcity and water ...
Published on by Water Network Research, Official research team of The Water Network
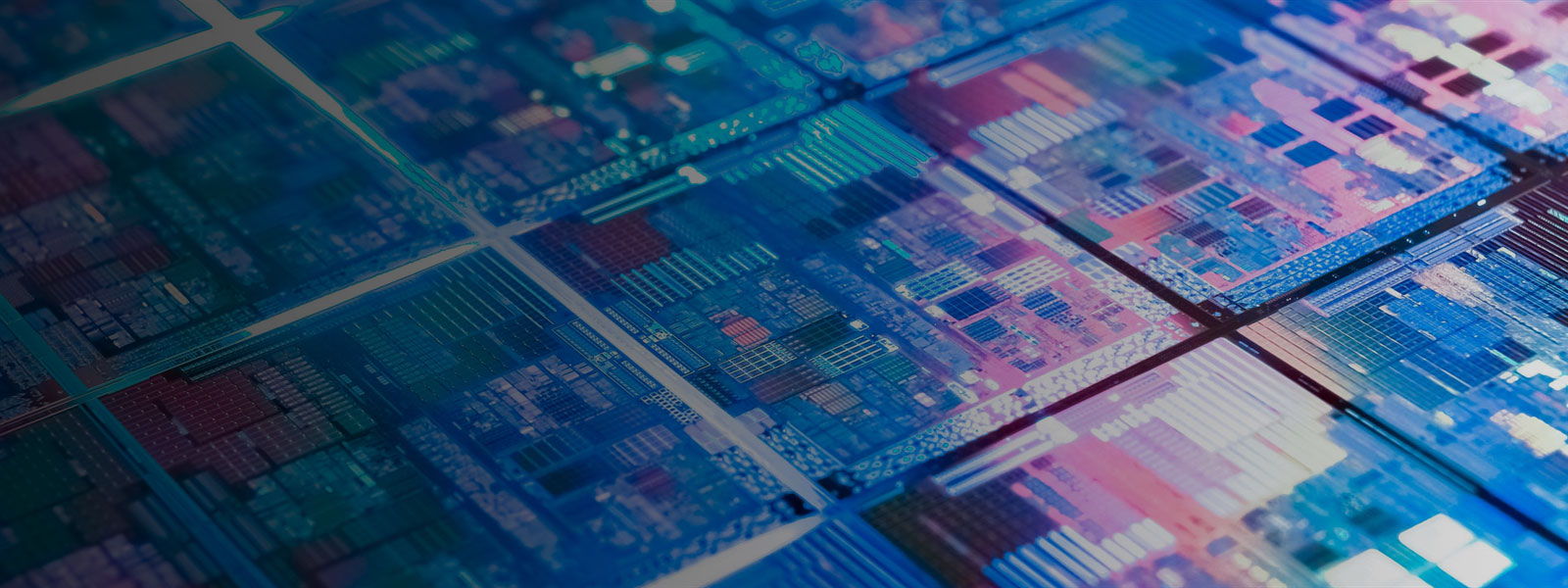
Communities across the globe are facing water scarcity and water management issues which are intensified in many regions by the impacts of population growth, urbanization and climate change. Developments in artificial intelligence (AI) open the door to transformative opportunities for heightened water management efficiency, with the potential for data and new technologies to become modern allies for water utilities to address longstanding issues, such as aging infrastructure and water loss.
Investment in digital water solutions is expected to grow 6.5 percent annually in the US, achieving an annual capital expenditure of US$10.8 billion in 2030. According to a 2024 Bluefield Research report, most of this investment is expected to be allocated to metering and customer management technology (41 percent), while other segments expected to receive large portions of this investment include network management (20 percent), work and asset management (18 percent) and plant management (18 percent).
How AI can improve water management
Some examples of the ways that AI can be implemented in water management include:
Smart water management
By analyzing data relating to weather forecasts, water availability, water usage patterns and population growth projections, AI systems can assist local governments in better understanding ways to optimize distribution networks and water allocation, particularly in an environment of water scarcity.
AI systems can also assist with the optimization of pumping schedules and adjustments to water flow and pressure to address actual water demands, thus minimizing water waste and enhancing network performance. For water systems, this results in reduced operational costs, including due to reduced energy consumption. For customers, the benefits include increased water service reliability and lower price increases because of the operational cost savings.
Predictive maintenance and leak detection and prevention
Operational efficiency in water treatment can be achieved by using AI to predict equipment failures and improve maintenance schedules. By analyzing data from sensors, equipment logs and historical maintenance records, AI can be used to identify patterns indicative of impending equipment and infrastructure failures. Similarly, and with the support of satellite imagery, AI can provide real-time monitoring of the conditions and performance of water pipelines.
Additionally, algorithms can be used to identify patterns or anomalies, which may indicate an actual or potential water leak or burst. These use cases of AI work to increase the longevity of infrastructure, minimize the chances of costly and disruptive unplanned downtimes and prevent significant water loss.
Energy consumption optimization in wastewater treatment
In the wastewater treatment sector, aeration—an important step in the water purification process—requires a significant amount of energy for pumping oxygen into basins to facilitate growth of the microbial population required for effective wastewater treatment. This stage of the treatment process can account for up to 60 percent of a wastewater facility's energy needs. By analyzing data associated with historical pollutant levels, wastewater flow rates, source and product water parameters, weather patterns and water use forecasts, AI can be used to continuously monitor and adjust, in real time, aeration rates in wastewater treatment facilities to achieve optimal levels. In doing so, AI allows wastewater treatment plants to minimize energy consumption and associated costs while ensuring an effective treatment process.
Precision agriculture
AI can be used to optimize irrigation practices by analyzing soil moisture levels, weather forecasts and crop characteristics, which can improve crop yields and minimize water usage. Given that the agricultural sector accounts for a significant proportion of global water consumption, the potential for the sector to develop more water-efficient processes could have a material impact on global efforts to conserve water.
As an example, in Arizona, the city of Tucson is already using AI to optimize water management processes. Specifically, AI is being used to identify patterns in historical pipe failures and evaluate data on soil, weather and land use to predict pipe breaks and calculate the associated likelihood and consequences of failure. This enables the city to make more focused and informed decisions about asset management and replacement, thus optimizing its maintenance budget allocations.
The paradox of implementation
While AI clearly offers numerous benefits for water efficiency, AI development has also had a large water footprint.
The direct effects of AI on water demand are seen in the water-intensive needs of the data centers required to train large-language AI models. It is estimated, for example, that a 1 MW data center requires up to 25.5 million liters of water annually to cool processors. US data centers are projected to require as much as 150 to 180 billion liters of fresh water in 2028. In tandem with the rise of generative AI and the global "arms race" to build out data centers to facilitate AI growth, we have seen a significant rise in the water usage of tech companies to keep up with market demands.
The rising water footprint of AI can be indirectly seen in the energy consumption of AI-associated infrastructure, as such energy also requires large amounts of water to be produced. Electricity consumption by AI servers is expected to exceed 150 to 300 TWh in 2028. Importantly, this does not account for the water consumption required to produce raw materials essential to certain data center components, such as the lithium typically used in the batteries that power data centers. For example, producing one ton of lithium salt is estimated to require two tons of water.
The increased water demand generated by rapid industrial development is being felt in numerous US cities. Earlier this year, Corpus Christi, Texas, enacted an emergency groundwater supply program allowing the city to lease well fields to provide surplus fresh water to the Nueces River, the region's main water supply. Water scarcity had been aggravated in recent years in the region due to prolonged drought and increased industrial sector growth—including a new lithium refinery and a new plastics manufacturing plant.
The water industry therefore faces a paradox between the water-intensive demands of AI-driven data centers—which often compete with local communities for fresh water supplies—and the potential for AI to improve water management processes in water treatment and distribution facilities and decrease consumption in water-intensive industries such as agriculture, thus increasing local communities' access to fresh water.
Attached link
https://www.whitecase.com/insight-our-thinking/ai-water-management-balancing-innovation-and-consumption?utm_campaign=Waterline%20Newsletter&utm_medium=email&_hsenc=p2ANqtz-97PoVIg5Iy2s9HeIznksis-youF-ZF2fdLUePhJZDweFwtqsBySI77pScni4otSOiRjCUPVvZZdwZOxskEWRHBgrREsA&_hsmi=354642472&utm_content=354642166&utm_source=hs_email&hsCtaTracking=63e41f91-b461-4d07-8d10-fc10dee97480%7C7d88eb69-188c-45c0-92f9-b2ccda93d164Taxonomy
- Water Efficiency
- Produced Water Treatment
- Industrial Water Treatment
- Industrial Water Managment
- Water Management
- Intelligent Networks
- Water Treatment Systems
- artificial intelligence
- Water Efficiency
- water treatment
- Water and Wastewater
- Water, Waste Water Chemical & Treatment
- Water and wastewater treatment
- Domestic Water Use
- Water Treatment Equip